What percent of academic papers published this year display similar sentences? And how is that percentage different across fields (it is much higher in, say, labor economics than urban economics, agent based or DGSE papers)? How far do computation concerns extend beyond the dedicated field and associated society created in 1995? To what extent the strategies today’s researchers develop to circumvent computation limits may, when repeated and spread throughout the profession, alter the type of models and techniques used? And how does the present situation compare to the constrainsts imposed to economists in the sixties or eighties?
How modeling choices in the forties to seventies were determined by computational constraints is one of the questions Judy Klein will adress in her new INET-funded project. She intends to show, for instance, how the science of economizing provided ways of thinking particularly suited to economists’ own attempts to deal with the scarcity of computational resources:
“The requirement of effective computation forced modelers to be mindful of their own scarce computational resources and bring rational thinking to their modeling production process –what Herbert Simon called procedural rationality. What emerged from all this were modeling strategies that adapted optimizing models of the problem to scarce information-processing capacities for the generation of a computable solution in the form of an algorithm that yielded a rule of action for operational use in the field. The large scale of the patronage and the logical imperative of the military client shaped applied mathematics in the USA in the 1940s-1960s into a science of economizing, and economists who could work with mathematical decision-making protocols and weave their economic way of thinking with a statistical way of thinking were selected, thrived, and passed on their skills to the next generation”
Longing for the stories she will tell in forthcoming workshops, I’m left with a few hints of how computational constraints in the postwar era affected not only economists’ modeling strategies but also their careers choice and practices. The correspondence between Franco Modigliani and Merton Miller in the early sixties shows how Miller’s decision to transfer to Chicago in 1962 was primarily directed, not only by a desire to move to an atmosphere more congenial to his rising, but by the computing resources the Chicago Graduate Business School offered, some both men badly missed at Carnegie. The 1962 report of the Computer Committee of the department of economics and the business school at MIT display similar concerns with how to use the 450 hours of computer time available per month and provide guidelines for trading between unsponsored research, “income producing activities” such as consulting, and teaching. Yet, it was because of the lack of trained faculty that a project to revamp teaching methods to incorporate more systematically the use of the computer was abandonned two years later. Such reform was eventually implemented in the mid sixties, after Ed Kuh had designed the TROLL software to help economists build and test econometric models. Kuh had divided econometric research into 8 macro-tasks, including a model input, model analysis, data input (mostly time series), estimation, estimation output and simulation phases. By the end of 1968, cross-sectional analysis, polynomial lag estimations, and stochastic simulations were enabled by the system.
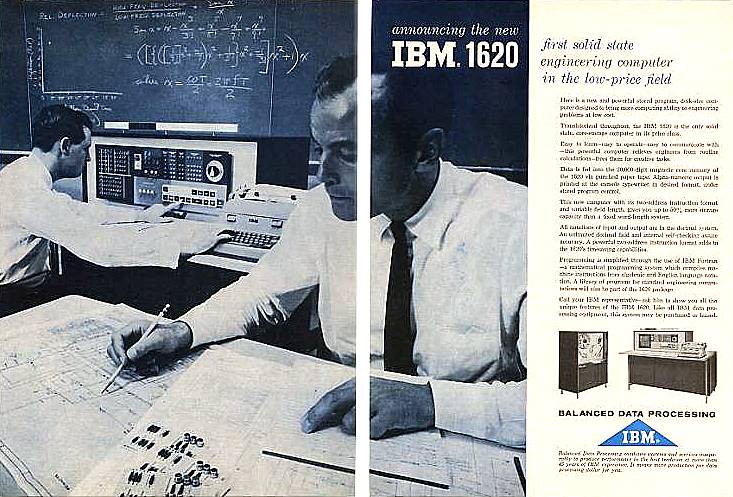
The IBM 1620 and IBM 7090 (here at the NASA) were the two computers used by the department of economics in the early 60s
These microhistories are consistent with the larger picture Lawrence Klein draws in an essay on the history of computation in econometrics (origins to mid eighties, reprinted here). In its first pages, the reader is brought back to an era (the thirties) when a wealth of research assistants used slide rules, the abacus, manually operated desk-top operators and punched card tabulators, when tricks such as the ‘Doolittle method” were used to take advantage of the symmetry of calculations in the estimation of least-square equations, when economists resorted to graphical fitting procedures, when matrix inversion took days of ‘progressive digiting.’
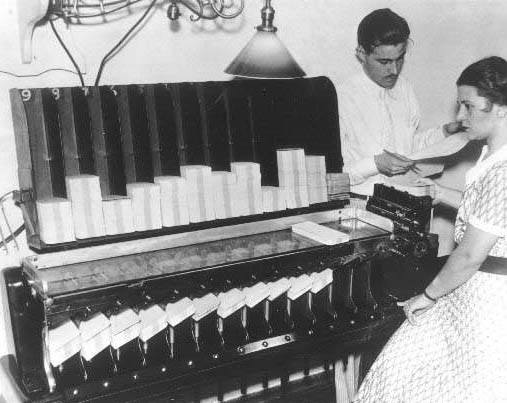
Type 80 IBM punched card tabulator
The Tinbergens and Kleins in charge of solving equations systems of 10-50 linear relations with lags paid great attention to the eigenvalues of their systems, used approximations and substitutions to specify their models as well as possible in advance of getting involved in heavy computational burdens. Typical issues such as Stigler’s diet problem (finding the minimum cost of a diet involving 9 dietary elements and 77 foods while meeting nutritional standards) took 120 man-days to compute by hand. Klein stresses how researchers lived with their data samples in these years, and understood them well from many angles. With the war focus on activity analysis, simplex methods were developed (by George Dantzig among others) to handle the computation involved in solving linear programming problems.
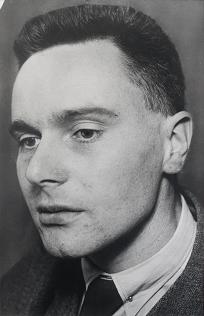
Jan Tinbergen
After the war, electronic computers were first used by Leontief for input-output analysis and by linear programmers. Klein then emphasizes how the use of the computer would not have spread without the pioneering efforts by Kuh, Morris Norman, Robert Hall and others to produce programs enabling various types of estimation, from least square to FILM, SLS etc… By the 1960s, Klein’s Brookings model consisted of more than 300 equations, not all linear (yet one block was linear in quantities with price given and the other linear in prices when quantities were given), and required computationally heavy matrix inversion for sets of simultaneous linear equations. Again, new methods of iterations were introduced to deal with these computation issues, and interestingly, the success of some of them (the Gauss Seidel method) owed as much to the efficiency of the algorithm itself as to the associated possibility to produce standardized graphs and tables readily usable by government agencies. From Klein’s account, one has the feeling that, in the sixties and seventies, economists were engaged in a computation race which involved the expansion of computation facilities and the improvement of computation methods on the one side, and, on the other, the new problems raised by developments such as stochastic simulations (in the wake of Irma and Frank Adelman’s study of model-genrated business cycles with random shocks), scenario analysis, sampling experiments using Monte Carlo methods, and the rise of cross sectional analysis enabled by the large family budget and labour market choices data compiled during and after the war. With the use of these large samples and of thousands of time series, data storage, update, and accessibility also became issues. With the development of telecommunications in the seventies, researchers such as Klein also set to combine and coordinate national econometrics systems and models (project LINK, based at Pennsylvania, involved 80 countries and 20 000 equations in the eighties).
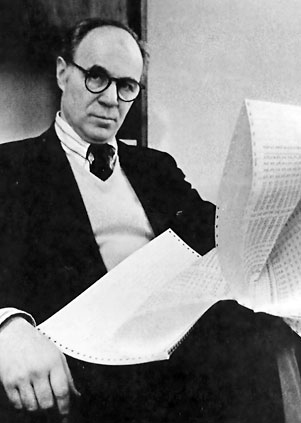
Lawrence Klein
Some comparison with the impact of computation on other sciences might also be relevant. Klein opens the aforementioned essay by explaining that he attended a 1972 symposium on computation in science at Princeton and was struck to hear that in many other fields (mathematics, natural science or psychology), specialists felt that the role of computer had not been decisive for scholarly development. This is however not the story Marylou Lobsinger tells in her study of how the evolution of computer science impacted the activities of the department of architecture at MIT. In the sixties, both design studio teachers and MIT faculty architects were trying to make architecture more scientific and more suited to the complex demands of a society riven with race and poverty issues and urban upheaval, and of the large city planning projects in which their discipline came to be embedded. They accordingly sought to transform their creative process into a rational mode of problem solving, one enabling better ways of collecting and analyzing data and interdisciplinary work. Rational decision making was to be achieved through the use of computer, Lawrence Anderson, Dean of the school of Architecture, stressed in 1968. Indeed, Lobsinger explains, “as built environment architecture could be represented numerically by means of statistics, set theory, algorithms or by means of geometric techniques able to calculate the optimum form for given conditions.” Architecture was experiencing an aesthetic revolution via technology, she concludes.
Although sketchy, these hints to the history of computation in economics help identify several “computation resources” whose scarcity and evolution influenced the course of scientific research in the postwar era: hardware (cost and computational abilities), the design of software, computer time for programming and running calculations (for research and education purpose), and computer specialists. Related questions include how institutions funded these resources, how access to computers and computer specialists was bargained between departments, disciplines, and research projects, how economic models were designed to fit the rudimentary facilities available in the fifties and sixties, and whether the increasing availability of computer power subsequently affected the course of economic research or its timing only (for instance, the lag between the theoretization of regression and their systematic incorporation into research publications).